牵引变电站人体入侵检测的研究开题报告
2020-04-28 08:04
1. 研究目的与意义(文献综述)
1、目的及意义(含国内外的研究现状分析)
1.1研究背景及意义
随着我国轨道交通特别是高速客运专线和城市轨道交通的快速发展,牵引供电系统发展迅速。强大可靠的供电是保证轨道交通正常运行的基本要求,牵引变电站是牵引供电系统中的重要环节,其安全运行是系统可靠运行的前提。
2. 研究的基本内容与方案
毕业设计希望针对牵引变电站,在前人研究行人检测和智能电站的基础上,对人体入侵这一具体问题展开研究。
因此,考虑将研究分为三个阶段来进行:采集数据、行人检测模型的实现、设计特征或指标区分正常的工作人员和闯入人员。
根据以往学者的研究与工作,行人检测的数据集一般采用加州理工大学的行人数据集(caltech predestrain)法国自动化所的数据集(inria).关于牵引变电站的人体数据的搜集,一方面来自于网络图片,其次来自于生产实习时的所拍摄的照片。
3. 研究计划与安排
第一周 | 查阅相关文献资料,明确研究内容 |
第二周 | 了解设计题目的各种要求,分析设计要点、难点 |
第三周 | 了解行人检测国内外的发展状况和研究现状,熟悉其基本的检测原理,完成开题报告 |
第四周 | 针对不同的功能模块查阅资料,熟悉系统各部分的检测原理,初步确定系统设计方案 |
第五周 | 搜集行人数据,并对自己采集的数据进行标注 |
第六周 | 完成行人检测中的特征提取部分 |
第七周 | 完成行人检测中的变形处理部分 |
第八周 | 完成行人检测中的遮挡处理部分 |
第九周 | 完成行人检测中的分类部分 |
第十周 | 完成各个部分算法的比较,选出适用于本次毕设的算法组合 |
第十一周 | 设计正常人员与闯入人员的区分特征,并对产生的算法进行有效性验证 |
第十二周 | 撰写毕业设计论文初稿,并交导师审阅 |
第十三、十四周 | 完成论文的最终定稿,交指导教师和评阅教师评阅,并准备答辩 |
第十五周 | 进行毕业设计答辩 |
4. 参考文献(12篇以上)
[1] DALAL N, TRIGGS B. Histograms of Oriented Gradients for Human Detection; proceedings of the Computer Vision and Pattern Recognition, 2005 CVPR 2005 IEEE Computer Society Conference on, F, 2005 [C].
[2] VEDALDI A, GULSHAN V, VARMA M, et al. Multiple kernels for object detection; proceedings of the Proc IEEE International Conference on Computer Vision, F, 2009 [C].
[3] WANG X. An HOG-LBP human detector with partial occlusion handling [J]. Procieee Intconfon Computer Vision Kyoto Japan Sept, 2009, 30(2): 32-9.
[4] VIOLA P, JONES M J. Snow D.: Detecting pedestrians using patterns of motion and appearance, F, 2003 [C].
[5] DOLLáR P, TU Z, PERONA P, et al. Integral channel features [J]. 2009,
[6] GAO W, AI H, LAO S. Adaptive contour features in oriented granular space for human detection and segmentation; proceedings of the Computer Vision and Pattern Recognition, 2009 CVPR 2009 IEEE Conference on, F, 2009 [C]. IEEE.
[7] WALK S, MAJER N, SCHINDLER K, et al. New features and insights for pedestrian detection; proceedings of the IEEE Conference on Computer Vision and Pattern Recognition, F, 2010 [C].
[8] FELZENSZWALB P, MCALLESTER D, RAMANAN D. A discriminatively trained, multiscale, deformable part model [J]. Cvpr, 2008, 8:(1-8.
[9] PARK D, RAMANAN D, FOWLKES C. Multiresolution Models for Object Detection; proceedings of the European Conference on Computer Vision, F, 2010 [C].
[10] YAN J, ZHANG X, LEI Z, et al. Robust Multi-resolution Pedestrian Detection in Traffic Scenes; proceedings of the Computer Vision and Pattern Recognition, F, 2013 [C].
[11] FELZENSZWALB P F, HUTTENLOCHER D P. Pictorial Structures for Object Recognition [M]. Kluwer Academic Publishers, 2005.
[12] DESAI C, RAMANAN D. Detecting Actions, Poses, and Objects with Relational Phraselets [J]. Bmc Veterinary Research, 2012, 18(1): 418.
[13] YANG Y, RAMANAN D. Articulated pose estimation with flexible mixtures-of-parts; proceedings of the Computer Vision and Pattern Recognition, F, 2011 [C].
[14] BOURDEV L, MALIK J. Poselets: Body part detectors trained using 3D human pose annotations; proceedings of the IEEE International Conference on Computer Vision, F, 2009 [C].
[15] ENZWEILER M, EIGENSTETTER A, SCHIELE B, et al. Multi-cue pedestrian classification with partial occlusion handling; proceedings of the Computer Vision and Pattern Recognition, F, 2010 [C].
[16] WU T, ZHU S C. A Numerical Study of the Bottom-Up and Top-Down Inference Processes in And-Or Graphs [J]. International Journal of Computer Vision, 2011, 93(2): 226-52.
[17] SHET V D, NEUMANN J, RAMESH V, et al. Bilattice-based Logical Reasoning for Human Detection; proceedings of the Computer Vision and Pattern Recognition, 2007 CVPR '07 IEEE Conference on, F, 2007 [C].
[18] DOLLáR P, APPEL R, KIENZLE W. Crosstalk Cascades for Frame-Rate Pedestrian Detection; proceedings of the European Conference on Computer Vision, F, 2013 [C].
[19] HOSANG J, OMRAN M, BENENSON R, et al. Taking a Deeper Look at Pedestrians [J]. 2015, 4073-82.
[20] TIAN Y, LUO P, WANG X, et al. Pedestrian detection aided by deep learning semantic tasks; proceedings of the Proceedings of the IEEE Conference on Computer Vision and Pattern Recognition, F, 2015 [C].
[21] SERMANET P, KAVUKCUOGLU K, CHINTALA S, et al. Pedestrian Detection with Unsupervised Multi-stage Feature Learning; proceedings of the Computer Vision and Pattern Recognition, F, 2013 [C].
[22] OUYANG W, WANG X. A discriminative deep model for pedestrian detection with occlusion handling; proceedings of the Computer Vision and Pattern Recognition (CVPR), 2012 IEEE Conference on, F, 2012 [C]. IEEE.
[23] OUYANG W, WANG X. Joint Deep Learning for Pedestrian Detection; proceedings of the IEEE International Conference on Computer Vision, F, 2013 [C].
[24] 王旭. 无人值守变电站智能视频监测系统的设计与实现 [D]; 华北电力大学, 2014.
[25] 张朝龙, 王硕, 梁凯, et al. 人眼视觉识别技术在变电站视频监控的应用 [J]. 电气应用, 2013, s1): 496-7.
[26] 武爱敏. 变电站视频中工作人员的识别 [D]; 华北电力大学(北京), 2017.
最新文档
- 芳香族化合物对哺乳动物急性毒性的QSAR模型:老鼠口 服LD50的研究外文翻译资料
- 基于风险的建设项目的非均衡定价检测模 型外文翻译资料
- 关于酒类饮料包装价值感知的实证分析外文翻译资料
- 注塑模具工业设计指导外文翻译资料
- 氨基官能化咪唑基氨基酸离子液体的CO2的吸收特性外文翻译资料
- 扩充旁观者的视角:探索增强现实技术用以提升在线服务体验的战略可能性’外文翻译资料
- 使用香港本地骨料的实心混凝土板中剪力钉的抗剪性能研究外文翻译资料
- 批判区域主义的展望外文翻译资料
- 不同层间阴离子的MgAl层双氢氧化物的DFT研究:结构、阴离子交换、 主客体相互作用和基本位点外文翻译资料
- 新型分层SiC纳米线增强SiC /碳泡沫复合材料:轻巧,超. 薄和高效的微波吸收体外文翻译资料
- 聚对苯二甲酸乙酯的激光烧蚀外文翻译资料
- 封项招标中评分参数之间的数学关系外文翻译资料
- 化学气相沉积制备ZrC-SiC复合涂层及共沉积机理的研究外文翻译资料
- 流体饱和岩石中剪切带的强度:膨胀和流体流动之间竞 争的非线性效应外文翻译资料
- 新兴经济体可持续房地产营销与实践面临的挑战以尼日利亚为例外文翻译资料
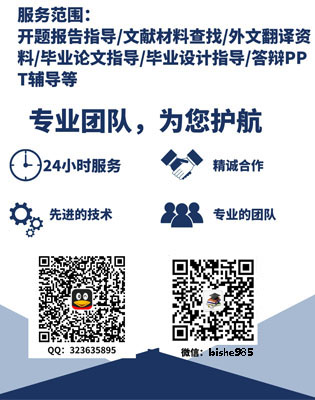